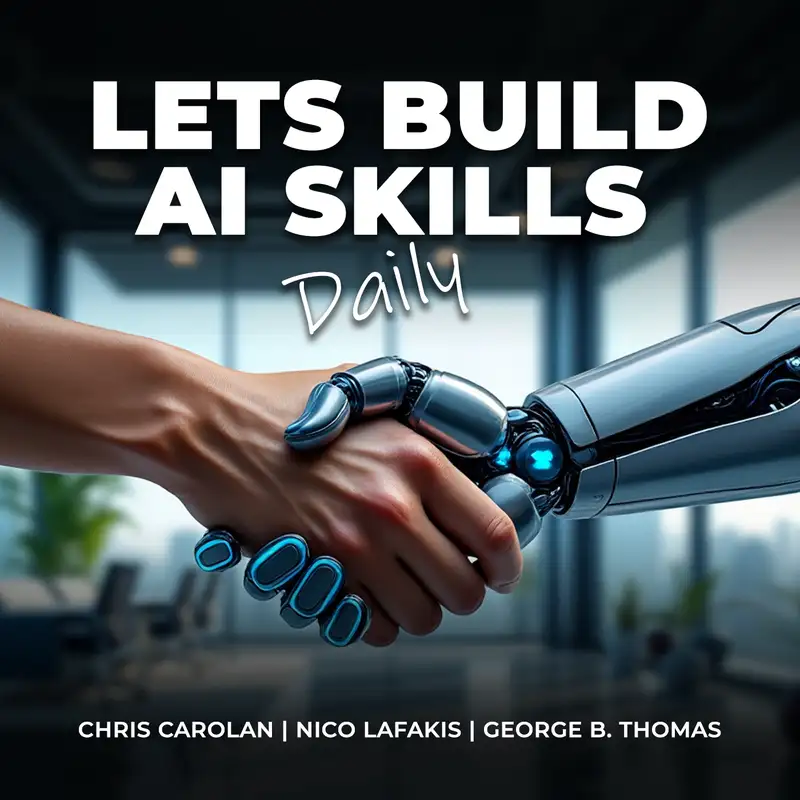
The AI S-Curve Evolving Models, and Computation Limitations
Welcome to wake up with AI, the podcast where human powered meets AI assisted. Join your hosts, Chris Carillon, Niko Lofakas, and George b Thomas as we dive deep into the world of artificial intelligence. From the latest AI news to cutting edge tools and skill sets, we are here to help business owners, marketers, and everyday individuals unlock their full potential with the power of AI. Let's get started.
Chris Carolan:Good morning. Happy Wednesday, November 13, 2024. It's time to wake up with AI. Here with Niko, George on personal assignment. But just me and Niko today, what do you what do you got, man?
Chris Carolan:How you feeling?
Nico Lafakis:I'm doing good. Got a lot per usual. There's exciting news. There's interesting news. There's really eye opening, mind blowing news.
Nico Lafakis:It's all over the place.
Chris Carolan:Some smelling salts, some visionary stuff as per usual.
Nico Lafakis:So Yep.
Chris Carolan:Wherever you wanna start, man.
Nico Lafakis:Well, I know that you and I have been have have actually, like, gone through and dug a little bit deeper into Claude and had some pretty in-depth conversations. And in in our chat, I dropped in a a video that was well, it was Lex Friedman's interview but it was with 2 different people from Anthropic. 1 was Dario Amadeh, the other I don't wanna butcher names except that he didn't actually Amanda Askel. I think it's I think it's Askel so I'm sorry if that's not right but Dario is one of the the chief builders. Amanda on the other hand is on the philosophical team.
Nico Lafakis:So she is much more in the realm of she actually is in charge of helping to build Claude's character and Claude's personality. Like the first half was about, you know, was with Dario and kind of where things are going with the company and everything. The second half was with Amanda Askel and it it was way more about, you know, philosophy behind what's going on with Claude and and how the thinking is built behind him and what goes on with that. So one of the clips that I thought was probably the most interesting was they got into a discussion about prompt engineering and I've talked about prompt engineering a lot. We've talked about it on this show a lot.
Nico Lafakis:That being the case, you guys know where my opinion lies on that? And, you know, I'm always interested in knowing, like, what the pro opinions are, the people who are actually building this stuff, what their opinions are on it. And I have heard several say that, like, hey. The model the models are at a point now where they know what they want. They know what the best instruction is.
Nico Lafakis:They can tell you how to best instruct them. That's why quad comes with a well, if you if you have a, paid subscription, it comes with a quad project that is specific to prompting for quad. And so I'm sure if you've used other tools, it has, like, prompt helping and stuff like that but I just thought that this clip in particular was really interesting because of the context and also the reasoning behind, like, prompting and, like, really the use case. I think this is almost like a perfect understanding of prompt engineering as a use case.
Chris Carolan:Yeah. And that's one of my favorite things right now is saying, I like what you just did there. What do I need to say next time to make sure it it happens like that again? Like, give me the prompt. Give me the instructions.
Chris Carolan:I'll add it in. I'll keep it over here wherever I gotta do because that is one of the most frustrating things, like, when you get gold and then next time you think you're doing the same thing, but all these other variables are in play and, you know, it's like, oh my god. So just take a step back and get help. Like, so often I go back into the chats, like, old chats, old projects and be like, hey. I really liked how this happened.
Chris Carolan:Like, give me the prompt framework to make that happen again.
Nico Lafakis:Right. What I've started doing is instead of having conversations, I've just built projects out of the conversations. And so I just whenever the output is really good based on whatever the project is that I'm working on, I just save that to the project. And so, like, as it goes on, I can always ask it to refer back to whatever it was. So I definitely suggest, like, building a prompt project basically.
Nico Lafakis:And, like, as you're going along and it's doing great prompts, that way when you run out of, you know, context window, you can always just spin up another one and it's already got the premise and it already understands the type of prompting you're doing and how how you like to have it done and all that. So I wanna play this clip and it's so interesting that you brought that up because this you're you're almost you're about to hear an echo.
Interview:There's a concern that people over anthropomorphize models, and I think that's, like, a very valid concern. I also think that people often under anthropomorphize them because sometimes when I see, like, issues that people have run into with Claude, you know, they say Claude is, like, refusing a task that it shouldn't refuse. But then I look at the text and, like, the specific wording of what they wrote, and I'm like, I see why Claude did that. And I'm like, if you think through how that looks to Claude, you probably could have just written it in a way that wouldn't evoke such a response. Especially, this is more relevant if you see failures or if you see issues.
Interview:It's sort of like, think about what the model failed at. Like, why what did it do wrong? And then maybe it give that will give you a sense of why.
Nico Lafakis:When you're working with these models and it seems as though you're you're having, like, bad feedback and you get, like, an answer that you weren't expecting or an answer that isn't the output that you wanted, a lot of times it can be because there wasn't some clear direction given or maybe it was that you didn't tell it what you didn't want to have happen or what you, you know, what you wanted to avoid. So it definitely, you know, again, back to Chris's point, it lends to what is it I really want. Right? It's it's like when you're asking for an image and you're trying to describe what you wanna see, you know, you could go with, like, oh, I wanna see a picture of a cat and, yeah, you know, whatever is to a 100,000,000 cats. Right?
Nico Lafakis:A 100 100,000,000 different ways to represent it or you can start getting more specific and, you know, you could say I wanna see a Bengal tiger with, you know, an overt amount of stripes that's hiding in a jungle, you know, at that mid morning or something like that, you know, eyeing and, gazelle in the in the distance. Totally different image, totally different, you know, output. Right? So we talked about it before that context is king and that's where you need to start out with things. And from there is where you can build out that great framework and that great response that you're looking for.
Nico Lafakis:But it's going to take iteration. It's going to take teamwork essentially. You're going to have to work with the model in order to produce the output that that you want. I thought, you know, I thought it would start there just like a little bit of a technical aspect of it.
Chris Carolan:And for those who may not know, the word anthropomorphize attributing human characteristics or behavior to some nonhuman thing. And we always come back to, like it's just like learning how to work with another human. It's just like when you delegate something else to a human, and they don't do it the way that you wanna do it. Did you communicate it well? Did you leave important details out?
Chris Carolan:Did they just mishear you because there was some random noise in the background that caused them to have to try and figure out what you're trying to say? Right? There's so many things that go into this kind of collaboration, right, with people, the same kind of things when you're working with, you know, Claude in this case, any AI tool, any software tool. This has always been the case. Like, did you give it the input that is required to get the output?
Chris Carolan:Right? And this is where the flexibility of no matter what you put in, it's gonna give you something versus erroring out. You know? It's a it's a learning curve, but when you learn when you learn that curve, when you go up the curve, like, it just becomes so powerful. And when you can take a step back to remember that and have some self awareness of maybe I didn't do what I was supposed to do in this situation.
Chris Carolan:Maybe it's not, you know, Claude doing something unexpected, and now I'm gonna run over to Reddit and complain about it. Just like any other tool, just like work with another human being, go back and forth with it, learn it, get better. Just get better.
Nico Lafakis:That doesn't necessarily lead into the the next story, but the next story is really, at least for me, it's profound and it's if you've been following progress, it's super profound. Dario will talk about in that video because it actually all of this news, it's so funny. Like, it all just kinda hit at the same time. And he didn't really talk about it, which kinda led me to believe, not necessarily that maybe he didn't know, but it wasn't something that he wanted to necessarily talk about because he has been a huge proponent when it comes to making these models better. He's been a huge proponent of this, scaling law and he thinks that essentially the more compute that you put behind the model, the better it's going to be.
Nico Lafakis:It's just a flat out rule. Well, that's wrong. It's turned from being a exponential curve upward based on compute to being an s curve. So what they've done and what OpenAI has has real realized and and sort of what this means is basically that the the the models are hitting a point at which the compute is starting to not matter and even the amount of data that's being shoved in isn't helping things. It's just getting marginally better now.
Nico Lafakis:It's not getting the exponential kick upward and this is happening across the board. This is happening at Google. This is happening at OpenAI. This is happening at Perplexity. It's happening at XAI.
Nico Lafakis:Everybody has hit the same wall and there was some expectation of a plateau, but it's interesting to think that, like, we're literally at the 2 year mark when we've hit this plateau and I think you guys remember me talking either last week or maybe even yesterday about the fact that we might be building, you know, that if you look at this from the lens of automotive, we built a steam engine of a model then we built like an inline form or, you know, like a 2 stroke engine then we built an inline 4 then a 6. Now we're kind of up to an 8 and it's super powerful, but all we've been doing is just adding more cylinders and increasing the the displacement of the engine. We haven't actually, like, tuned anything. We haven't changed the blowers. We haven't, like, done anything with fuel injection.
Nico Lafakis:We haven't even put, you know, any sort of, like, intake on it. We haven't done any exhaust, like, nothing. Right? So now we're doing that and that is the next, essentially, the next leg of the s curve. So instead of these being, like, exponential growth, but so what's interesting is, like, there is an exponent of growth, like, you could see between 34 o.
Nico Lafakis:Right? So that's a huge huge jump. All it could do is keep repeating itself over and over and everything was a deep dive and everything was, you know, a discovery and everything was, you know, the same same stuff over and over again. It was insanely difficult to work with. Now you have, you know, quad 35 or, you know, chat 01 preview or 4 o.
Nico Lafakis:It's night and day. It it like, it's not even it's not even worth comparing it to. You know, 3 doesn't even have the capability for upload and and data analysis or any of that, like, it's not even close. 3 is a kindergartner. 4 o is like a college student, like a graduate college student.
Nico Lafakis:So that's the leap in 2 years. What's after 4 year grad college? Master's and PhD. Well, Claude is already at a post like higher than PhD level in at least three areas of study. I think it's actually 5.
Nico Lafakis:GBT 0 1 is 3 year 3 PhD levels of study. So, we're almost, I mean, we've already passed masters in most categories. There are some categories like math which are, you know, just very difficult oddly enough for the model but it's because the model is based on language and not based on like doing mathematics, basically. You can guess where we're going to be. But the height of this s curve is AGI at just a pure compute mode.
Nico Lafakis:If you want to put this into perspective like what's about to happen between the two differences, like what they're working on is inference of the model. So, now they're going to be fine tuning is the reasoning through the data, the understanding of the data. There's gonna be a lot more post training that goes on. A lot more pre training that goes on. What does this look like?
Nico Lafakis:It looks like imagine that you just knew all of the things that there were to know, but you didn't really have as much of an understanding of the why and the how of these things go together. You just know these things and if somebody asks you about them, you can tell them, but you can't really, on your own, put new ideas together. You can't really, on your own, formulate things for yourself. You can't, like, the difference being like, if I wanted to talk to a current model in some type of bipedal form, you know, robot around my house, I'd have to say, hey, go to the I'd have to tell it the steps, the chain of thought. I'd have to say, hey, go to the refrigerator, open the door, reach down to the 2nd shelf, grab the carton of eggs with a light amount of force, bring that out, put it up on the counter, close the refrigerator door, come back to the counter, open the carton of eggs, hand me one egg.
Nico Lafakis:The level we're about to get to and and kind of are at at this point, get me the eggs from the refrigerator and everything in between is already understood and already done. We're fine tuning that aspect of it. Almost you could consider this the ESP aspect of it. We're fine tuning the ability for it to best understand what it is that we want with the initial ask. And not only that, but because of that, the ramification of it best understanding is again reasoning.
Nico Lafakis:And if it can better reason, then it can essentially think better. It can think smarter, can think through problems better than better than we can. So like the plateau of this level is AGI. AGI and agents. Right?
Nico Lafakis:We're gonna we're about to have things that are we're gonna be able to I'm actually gonna show 1. I've been messing around with something. It's not AGI. This is it's a really it's an insult to even compare the 2. I ran across this yesterday.
Nico Lafakis:It's a application from Replit and if you don't know, Replit is a online platform that you can use in order to launch any type of software that you want for testing. And recently, I've been messing around with no code stuff like crazy. I've been in agent AI, I've been in Zapier, I've been in every no code based agent you could possibly think of. This one destroys all of them. All I've been doing up until now, you can kind of see what, like, what's going on here.
Nico Lafakis:This application to build out customer journey stages, and I can change the name of the stage, change the description, conversion rates, and then when when I'm done with this, I can go over to analytics. Well, you know, how did I get this? How did I get to this? I literally just went to replit and I just gave it a prompt. I just want a web based customer journey visualization tool helps with business mapping, helps businesses analyze their customer conversion paths through interactive flowcharts and and funnels.
Nico Lafakis:Here's the core features I want. Here's the style I want it to be in. Absolutely. Let's get to work. I'll help you build out that thing.
Nico Lafakis:We'll build the initial prototype, we'll implement advanced analytics dashboard, and then we'll enable the, journey version history. Yeah. Let's go. So it builds out the initial stuff. Then it actually put up a test for me before this that was just, hey.
Nico Lafakis:Play around with the interface. See if it works. Yeah. It's working. Alright.
Nico Lafakis:Well, I'll fix, you know, what's going on with that. You know, I could see that there's a time stamp error. So it went ahead and and fixed it. I didn't even catch the time stamp error. It caught it and was like, yeah.
Nico Lafakis:You know what? Let me go back and fix this thing. So then can you try updating the journey stages? No errors present when doing the updating, saving, or creating new ones. Cool.
Nico Lafakis:I'll build out the rest of the advanced analytics dashboard and then can you navigate the analytics dashboard and confirm that it loads with the tabs for overview stage analytics and trends. So, I can first, I just wanted to see if the template aspect of this works. Not kinda not yet, but that's fine. I'm sure it'll oh, that's that's funny. I didn't even notice that it just, by default, built in built in a, dark mode to it.
Nico Lafakis:I don't even know. What's funny is it initially showed it to me in dark mode as if it understood that it was already working in a dark mode setting from Replit. You know, I don't know if that's the case, but I just thought it was cool. So yeah. So we can see, like, stage tran you know, stage analysis, trans overview.
Nico Lafakis:Clearly, this isn't hooked up to a CRM, but it could be. Essentially, what you're looking at is the potential for me to build out, oh, I don't know Marketo, and then just I could probably I didn't wanna get, you know, I didn't want us to get in trouble, but I could honestly say something. Probably say something like, hey, can you build something out that is that mimics the Marketo platform that I can use to plug in an API key to my CRM and and funnel analytics data into it? So Yep. As I can.
Chris Carolan:And I'd say, like, another approach people could take is, like, getting help understanding why, especially if you're somebody who's never used systems like this before. Because all these systems, like, I remember back when it was my decision in 2017 to do marketing automation for the business. Right? I signed up for Marketo. Didn't go well in the 1st 30 days.
Chris Carolan:Found HubSpot. The difference between those two systems as a marketing automation platform, vast. So this is one of those, like, human things that if you can take a step back and say, no. Let's not. Both systems have had their, you know, weaknesses over the years.
Chris Carolan:So instead, like, why do I even need to use this system? And then all those whys that pop out, give it to the AI, see what kind of system it can create based on all those goals. You know, because so much of it's based on the data that you have access to. So it's like, hey, I wanna do this. Here's the spreadsheet, the spreadsheet, the spreadsheet, the spreadsheet.
Chris Carolan:Let's get after it. You know, people talk about focus and expectations early on and finding the quick wins. And, like, instead of making it about what people feel like they want, you know, show the data and show the objectives and see what gets built out. That's pretty exciting to think about.
Nico Lafakis:What you're looking at, we've talked about it before, but the reason I wanted to show this is that specifically to show people that this is what that wow, naturally intuitive, this is what that environment looks like. This is what that world looks like of 0 cost production. You know how much it costs me to start building this app out? $25 a month. For $25 a month, I can now go in here and generatively, no coding, I can generatively build out applications all day long and even if they don't come out perfect, I can sit here and edit and work with it and Replit is a code based platform.
Nico Lafakis:So, believe me when I say that its model, extremely fine tuned to coding and is probably right up there with Claude in terms of being able to code on its own. So realistically, as you can see, full stack app. Hate to say it. Been talking about it. Been mentioning it.
Nico Lafakis:Tried to warn you about it. You are looking at an automated AI driven full stack developer. All of these files over here, it is generating on its own. Everything that it needs in order to run the application that it's building, it is building out on its own. I'm not doing anything.
Nico Lafakis:It's just going through it. It'll eventually get to the next step. It'll ask me to test it, then it'll ask me if I need feedback. This is what an agent is. This is an agent.
Nico Lafakis:It is going to do hours worth of work. I don't know how long it's gonna take. Personally, don't care. All I care about is getting my application done and that just entails it doing the work and if it needs to come back to me for verification. We were just talking about this yesterday.
Nico Lafakis:Like, this is the visual example of that. I'm able to just let this go do its job. I can sit back do nothing and it'll just prompt me whenever, like, hey, ran into this problem or hey, here's the color scheme. Do you want to change it? You know, it'll just ask me personal preference in testing.
Nico Lafakis:Otherwise, I don't have to touch anything. I don't have to do anything. Right? So yesterday, we were talking about using quad to level up and advance your ability of being able to do custom coded actions. Today, 24 hours later, we're talking about a generative AI application that is a full stack developer where you can just ask it for an application and it'll build it out for you automatically.
Chris Carolan:That's power. And it's like I have no dev experience at all. I just what things come to mind is like when I run into issues and and the dev's like, oh, I had to add this JavaScript file or I had to connect this dot with that dot, and now it's good to go. Just so many of the challenges. But, also, like, if you're developing product right now, just take care, like, in what you're deciding to develop because this is where and I think you might have started with this, this moment where there's perfect information available.
Chris Carolan:And I kinda think of it like the next step. So perfect information is like everybody's PhD level, let's say. Right? We know the challenge of university is often people get out of university, have no no idea what the hell to do in the real world. It's all theory based knowledge.
Chris Carolan:They can't apply it to anything, and that's kind of, like, when we think of this next level. Right? Once it once perfect information exists, and I'm glad I'm glad we've got there to, like, where you don't need more compute to get to get the perfect information. Basically, it's just more compute makes you overthink it like we do as human beings all the time. Like, don't sell past clothes, like, all that stuff.
Chris Carolan:Right? Now, you have this information set getting to the moment of perfect application of it. Right? Sounds like AGI or or how people talk about it and, you know, know, in one of the videos you shared, it talked about the coffee. It was like the coffee problem or something like that where you can just tell the robot to go into a house that's never been in before and make coffee without giving all of the instructions that you mentioned before.
Chris Carolan:That's like a personal level of basically agentic behavior. Like, it can know, like, how do it how to evaluate the house, like, what a refrigerator looks like versus what the cupboard looks like and, you know, okay. I expect it to be here, not there. Now I'm gonna look over here. Like, all this decision making that has to happen, which is fun for me.
Chris Carolan:It's always fun to be a little introspective and think about, oh, man. We take a lot of this stuff for granted. But when you hear things like how many decisions we have to make in a day, right, and decision fatigue and all this stuff. We talk about cognitive burden and overload. Like, we are doing all this stuff subconsciously all the time.
Chris Carolan:And those are the like, that's where it's not there yet. When it gets there, it will be amazing, but this is also why it's not there yet. Like, since we don't even know that about ourselves very well, and we're the ones training it, it's gotta overcome some of our shortcomings to get there. But yeah. So I think, like, the fact that we've hit this part of the s curve is definitely fun to think about from a perspective of, like, as long as it everybody's saying, like, more compute, more energy, more this, more that.
Chris Carolan:It's like, okay. When are we gonna get to the moment of it starts to get more efficient and starts to go down that hill? Sounds like if they've confirmed that you don't need more compute to get past this point. You know, maybe we're closer to that, and it's just about refining and, you know, developing the rest of the systems, right, outside of the engine, basically.
Nico Lafakis:Yeah. I think that it's definitely going to be a case where and I think it's gonna be very soon. I don't think that it's going to be something where it's gonna be years from now that we see the end result of this. I think it's going to be, like, a year from now. If you think back, it wasn't even very long ago that Sam said that he's very confident that we would have artificial super intelligence within 1,000 of days.
Nico Lafakis:I don't think that it's going to take that long, if I'm being honest. I think that it's going to be shorter than that. So, like, this is what the current sort of s curve looks like, right? So we had like g p t 2 which was like nothing and now we're getting towards this level of Orion, Right? So this is the peak of the s curve.
Nico Lafakis:If you're looking at it properly, it's like, again, this is probably where we come into AGI. You know, it could be, you know, 01, 02, 03, but I honestly think that it's right at this point, this initial inflection. So whenever we make this first transition is when there's going to be, like, a really greater understanding of what's going on. The fact that this says 2526, not for nothing. This is, I think from, AI Advantage.
Nico Lafakis:You can't look at so this is where the exponent comes in. I think. I know this seems really weird. I mean, it is still an s curve, but I think it's maybe more that this area is softer. I think it's a very short amount of time that we live in this can't figure it out stage.
Nico Lafakis:Again, if we're already using AI to develop better hardware, there's no doubt in my mind that we're using it to develop better software and at least to give us the ideas of how we could make it better which is why I think we're headed down the road of like, oh, just better training. You know? And back like what I was saying before was, you know, you could have a brain that's full of tons of data. The only way you make it super smart is if you fine tune it and if you get it to understand how all that works together. So getting it to understand how all the data works together, that's not gonna take as long.
Nico Lafakis:It's probably only going to take a year. I really do think like within this next year, like if 0 one is performing where it's at now and it's not quite AGI, this this sort of marker is is off because we're at the end of 24. So you can see like if I were gonna plot it, I'd say very beginning of next year, March March April, like March, probably March, April, we'll at least get an example and sort of get, like, a a hint of, like, hey. Here's here's where we actually, like, are getting out.
Chris Carolan:Yeah. I think the only safe bet is that it's going to happen faster than anybody thinks. And if somehow you're still sleeping on AI, it is time to wake up with AI. Have a great day, everybody.
Intro:That's a wrap for this episode of wake up with AI. We hope that you feel a little more inspired, a little more informed, and a whole lot more excited about how AI can augment your life and business. Always remember that this journey is just the beginning, and that we are right here with you every step of the way. If you love today's episode, don't forget to subscribe, share, and leave a review. You can also connect with us on social media to stay updated with all things AI.
Intro:Until next time. Stay curious, stay empowered, and wake up with AI.
Creators and Guests
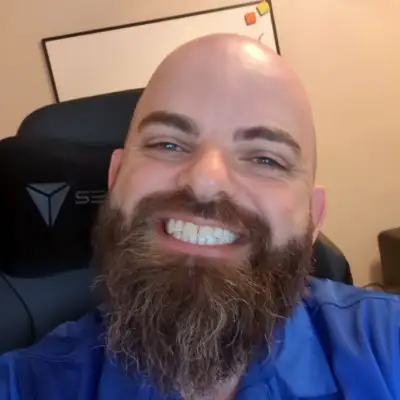
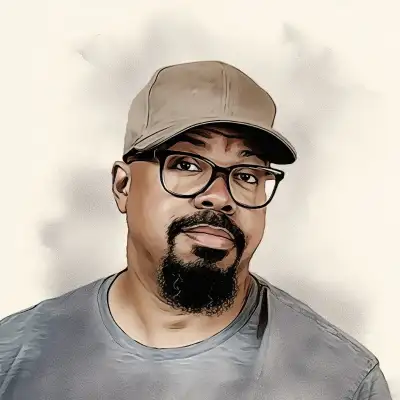